Note: I wrote this post a few years back and left it lying in the draft pile, unable to come up with a satisfactory conclusion until earlier this year. Our forecast calls for snow tomorrow (something those of us who live in RVs would rather not see), so a post about precipitation and weather prediction might be apt. –TAW
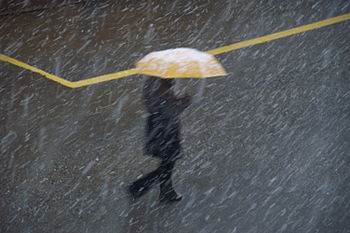
[This post begins our hard look at Chapter 6, “The Hard Stuff” in Carrier’s Proving History. — specifically, the section entitled “Bayesianism as Epistemic Frequentism.”]
In the 1980s, the history department building on the University of Maryland’s College Park campus had famous quotations painted on its hallway walls. Perhaps they still do.
The only quote I can actually still remember is this one:
“The American people never carry an umbrella. They prepare to walk in eternal sunshine.” — Alfred E. Smith
I used to enjoy lying to myself and say, “That’s me!” But the real reason I never carry an umbrella is not that I’m a naive Yankee optimist, but rather because I know if I do, I will leave it somewhere. In this universe, there are umbrella receivers and umbrella donors. I am a donor.
Eternal sunshine
So to be honest, the reason I check the weather report is to see if I should take a jacket. I’ve donated far fewer jackets to the universe than umbrellas. But then the question becomes, what does it actually mean when a weather forecaster says we have a 20% chance of rain in our area this afternoon? And what are we supposed to think or do when we hear that?
Ideally, when an expert shares his or her evaluation of the evidence, we ought to be able to apply it to the situation at hand without much effort. But what about here? What is our risk of getting rained on? In Proving History, Richard Carrier writes:
When weathermen tell us there is a 20% chance of rain during the coming daylight hours, they mean either that it will rain over one-fifth of the region for which the prediction was made (i.e., if that region contains a thousand acres, rain will fall on a total of two hundred of those acres before nightfall) or that when comparing all past days for which the same meteorological indicators were present as are present for this current day we would find that rain occurred on one out of five of those days (i.e., if we find one hundred such days in the record books, twenty of them were days on which it rained). (Carrier 2012, p. 197)
These sound like two plausible explanations. The first sounds pretty “sciency,” while the second reminds us of the frequentist definition of probability, namely “the number of desired outcomes over the total number of events.” They’re certainly plausible, but do they have anything to do with what real weather forecasters do?
Recently, I came across an article on this subject by a meteorologist in Jacksonville, Florida, written back in 2013. He even happened to use the same percentage. In “What does 20% chance of rain really mean?” Blake Matthews writes:
When a meteorologist says that there is a 20 percent chance of rain, that is not a ”cover your rear” percentage. It usually means that the atmosphere is generally stable but there’s just enough of a particular ingredient (i.e. moisture, heat, lift) to squeeze out a shower of [over?] a very limited area. That doesn’t mean the weatherman said it wouldn’t rain. It just means that the chance of you being affected by it is very low. (Matthews 2013)
All right, so I gather if the value is at 20%, we can leave our umbrellas at home without too much risk of getting wet. However, I still don’t think I fully understand what they really mean and what they’re basing the number on, but help is on the way. Matthews asked George Winterling, their hurricane expert, to explain further:
First, I would point out that it does not mean that 20 percent of the area will get rain. There was a definition originally given by the National Weather Service (when it was the U.S. Weather Bureau) that stated that it was the chance that at least .01 inches of rain will fall at a single point in the forecast area. And considering the where and when that convection will produce rain, it’s a gamble (probability) that rain will be produced in any of the hundreds of clouds that pass overhead. (Matthews 2013, emphasis mine)
Popping the bubble
That scratches out Carrier’s first guess as to what 20% chance of rain means. It isn’t a terrible guess, since it almost matches the old U.S Weather Bureau definition. (It became the National Weather Service in 1970.) But I still don’t have a handle on what it means. Matthews writes:
Rain chances are oftentimes categorized. The National Weather Service does this routinely with the terminology ”chance PoPs” and ”likely PoPs” — PoPs being an acronym for ”Probability of Precipitation.”
Chance PoPs are the garden variety PoPs. This usually is the 10 to 30 percent range. In this percentage, a quiet afternoon is likely but there is some atmospheric condition, like the sea breeze, that may have just enough energy to ring out a shower somewhere. These are the showers that hit your neighbor’s yard but not yours. (Matthews 2013, spelling altered, emphasis mine)
Now that we know the official term, let’s go to the source, the National Weather Service at http://www.weather.gov. It’s one of their frequently asked questions. I wouldn’t doubt that it’s one of their most frequently asked. Here’s what they have to say:
What does this “40 percent” mean? …will it rain 40 percent of the time? …will it rain over 40 percent of the area?
The “Probability of Precipitation” (PoP) describes the chance of precipitation occurring at any point you select in the area.
How do forecasters arrive at this value?
Mathematically, PoP is defined as follows:
PoP = C x A
where “C” = the confidence that precipitation will occur somewhere in the forecast area, and where “A” = the percent of the area that will receive measurable precipitation, if it occurs at all.
So… in the case of the forecast above, if the forecaster knows precipitation is sure to occur (confidence is 100%), he/she is expressing how much of the area will receive measurable rain.
PoP = “C” x “A” or “1” times “.4” which equals .4 or 40%.
But, most of the time, the forecaster is expressing a combination of degree of confidence and areal coverage. If the forecaster is only 50% sure that precipitation will occur, and expects that, if it does occur, it will produce measurable rain over about 80 percent of the area, the PoP (chance of rain) is 40%.
PoP = .5 x .8 which equals .4 or 40%.
In either event, the correct way to interpret the forecast is: there is a 40 percent chance that rain will occur at any given point in the area. (National Weather Service FAQ, emphasis and reformatting mine)
In a PDF document from the NWS, the author claims that PoP is “one of the most least [sic] understood elements of the weather forecast,” unwittingly demonstrating why scientists often have trouble communicating to the public.
To summarize, the probability of precipitation is simply a statistical probability of 0.01 inch or more of precipitation at a given area in the given forecast area in the time period specified. Using a 40% probability of rain as an example, it does not mean (1) that 40% of the area will be covered by precipitation at given time in the given forecast area or (2) that you will be seeing precipitation 40% of the time in the given forecast area for the given forecast time period. (NWS, Weather Education)
Instead, they say, it’s a combination of confidence multiplied by area coverage, both expressed as decimal values. Incidentally, by this time you may have noticed that Carrier’s second guess must also fall by the wayside — PoP is not the measuring of frequencies in record books.
Meanwhile, across the pond
In the UK, by contrast, the Met Office reports the probability of precipitation not as a combination of confidence times area, but as a simple percentage. (Note: I presume that the confidence of the forecast is “baked in” to the final number, but not shown to the public because it really doesn’t affect the final “message.” If anything, partially showing the work that goes into building the PoP seems to confuse the average person.)
So what does a PoP of 10% mean? This means that there is a 1 in 10 chance that precipitation will fall during this period. Another way of looking at this probability is that there is a 9 in 10 chance that it will stay dry. Similarly, a PoP of 80% means an 8 in 10 chance that precipitation will fall, and only a 2 in 10 chance that it will remain dry. (Met Office)
That’s pretty clear. And to get that probability value, they plug current observations into a computer model and run the simulation over and over.
To estimate the uncertainty in the forecast we use what are known as Ensemble Forecasting. Here, we run our computer model many times from slightly different starting conditions. Initial differences are tiny so each run is equally likely to be correct, but the chaotic nature of the atmosphere means the forecasts can be quite different. On some days the model runs may be similar, which gives us a high level of confidence in the weather forecast; on other days, the model runs can differ radically so we have to be more cautious. (Met Office, emphasis mine)
So now we have a bit of insight into why a meteorologist might have low confidence in a forecast. The conditions are so volatile that the confidence range rather large.
Ensemble forecasting and Bayesian model averaging
To be sure, we’re talking about extremely large amounts of data and some pretty complex calculations. But people often depend on the forecast outputs to avoid catastrophic events like floods, storms, tornadoes, and hurricanes. So these three tasks: (1) making sense of the data, (2) coming up with accurate predictions, and (3) communicating them effectively to the public are not trivial matters.
Getting back to ensemble forecasting, meteorologists gain confidence in their predictions by running the same simulations again and again but with tiny changes in the initial data. These multiple forecasts are called an “ensemble.” Unfortunately, it turns out conventional methods for comparing the ensemble forecasts can produce inaccurate results. In the video below, Adrian E. Raftery, a professor of statistics and sociology at the University of Washington, explains how Bayesian Model Averaging can improve those results. He also highlights those three tasks above with respect to weather forecasting. It’s a bit technical, and sometimes the audio is hard to follow, but overall I think it’s well worth watching.
[youtube=https://www.youtube.com/watch?v=4TMYFWG_c2M]
Conclusion
If your takeaway from this post is that I’m bashing Carrier for dreaming up definitions of PoP, you’re missing the point. Instead, what he missed, at least in my estimation, was an opportunity to note the confluence of observational science, computer modeling, statistics, and psychology. Specifically, how do we use science and math to help us predict future events, and then how do we express those findings to the public in ways people will understand them?
These questions apply in the social sciences as well — including disciplines in which we’re not predicting events in the future, but evaluating the likelihood that something occurred in the past. In the field of forensics, for example, experts need to collect data, evaluate the information gained from that data, and then convey its meaning to non-experts.
Tim Widowfield
Latest posts by Tim Widowfield (see all)
- How Did Scholars View the Gospels During the “First Quest”? (Part 1) - 2024-01-04 00:17:10 GMT+0000
- The Memory Mavens, Part 14: Halbwachs and the Pilgrim of Bordeaux - 2023-08-17 20:39:42 GMT+0000
- The Memory Mavens, Part 13: The Purpose of Halbwachs’s La Topographie - 2023-05-22 16:15:00 GMT+0000
If you enjoyed this post, please consider donating to Vridar. Thanks!
Tim – thanks for this.
When I read R. Carrier’s interpretation of what “20% rainfall” means I also agree with your plea of dissonance.
But I’ve always had an understanding on it, I had never had it confirmed. But now it seems my understanding might be different from the official definitions you have above, and that confuses me a bit. May be I need to re-read them. My understanding has been complex as detailed below.
[Chance of Conditions Occurring for all possible range of types of rainfall to occur for significant duration over a given region]
So let’s say there are 20 possible rainfall types
For type 1 – mild spitting
The conditions that historically have led to mild spitting are x,y,z.
Currently the conditions are delta x, delta y and delta z.
The statistical chances of each delta moving closer to the conditions can be predicted based on directions of currents and flows, time of day and season and what is happening outside the target window, etc. This creates a value from which a normal distribution for each parameter x, y and z can be applied. A predictor is found.
This is the chance of mild spitting.
The same is done for type 2 rain and then type 3 rain and so on …
The chance of any rain falling more than a nominal amount, greater than a nominal duration for that footprint – that would equal the greatest value obtained from the 20 types. I would say that torrential rain is much less probabilistic than mild spitting, because the conditions for torrential rain are more specific than for mild spitting, and as the chances of of torrential rain increases all the lesser types of rainfall become higher making the – “mild spitting” inevitable. So I used to think they report the weather based on the best case scenario, not sure if this is the same as above, but it could just as well be the chance of the mid-range of rain type.
That is, if there is 20% chance of a proper hour long period of rain, this is taken from a set of figures that suggest may be 90% chance of mild spitting and may be 5% chance of a torrent to occur in the same region at the same time. The percentages are taken from the chances of the conditions for rain to occur to occur.
I think they should be though if not.
But for whatever reasons, the UK definition seems very close to the simplified Bayesean/Carrier/layman definition?
I don’t think it’s all that close to Carrier’s definition(s).
UK: “This means that there is a 1 in 10 chance that precipitation will fall during this period.”
Carrier, you will note, gives two incompatible and incorrect definitions:
(1) “. . . either that it will rain over one-fifth of the region for which the prediction was made”
(2) “. . . when comparing all past days for which the same meteorological indicators were present as are present for this current day we would find that rain occurred on one out of five of those days.”
His definitions are whimsical, but they don’t conform to real weather forecasting. Both the US and the UK rely on computer modeling and the close monitoring of conditions (temperature variations, wind speed and direction, humidity, etc.).
You can find more information here:
https://whyitrainedtoday.co.uk/index.php/2018/05/31/a-tale-of-a-statistician-without-an-umbrella/
I think both of Carrier’s definitions are equivalent.
If you’re in the target region, saying there’s a 1/5 chance you would experience rain is the same as
1. there’s a 1/5 chance of rain in that region; and
2. rain will fall with 100% probability over 1/5 of that region.
Either way, your chance of experiencing rain is 1/5.
There are all kinds of vagaries involved in “common sense” probabilities. When a weatherperson foresees a 1/5 chance of rain, either or a combination of these cases is meant.