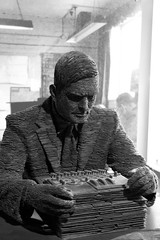
Misunderstanding a theorem
Over on New Oxonian, Hoffmann is at it again. In “Proving What?” Joe is amused by the recent Bayes’ Theorem (BT) “fad,” championed by Richard Carrier. I’ll leave it to Richard to answer Joe more fully (and I have no doubt he will), but until he does we should address the most egregious errors in Hoffmann’s essay. He writes:
So far, you are thinking, this is the kind of thing you would use for weather, rocket launches, roulette tables and divorces since we tend to think of conditional probability as an event that has not happened but can be predicted to happen, or not happen, based on existing, verifiable occurrences. How can it be useful in determining whether events ”actually” transpired in the past, that is, when the sample field itself consists of what has already occurred (or not occurred) and when B is the probability of it having happened? Or how it can be useful in dealing with events claimed to be sui generis since the real world conditions would lack both precedence and context?
I must assume that Joe has reached his conclusion concerning what he deems to be the proper application of Bayes’ Theorem based on the narrow set of real-world cases with which he is familiar. He scoffs at Carrier’s “compensation” that would allow us to use BT in a historical setting:
Carrier thinks he is justified in this by making historical uncertainty (i.e., whether an event of the past actually happened) the same species of uncertainty as a condition that applies to the future. To put it crudely: Not knowing whether something will happen can be treated in the same way as not knowing whether something has happened by jiggering the formula.
Different values yield different answers!
I’m not sure what’s more breathtaking: the lack of understanding Hoffmann demonstrates — a marvel of studied ignorance — or the sycophantic applause we find in the comments. Perhaps he’s getting dubious advice from his former student who’s studying “pure mathematics” (bright, shiny, and clean, no doubt) at Cambridge who told him:
Its application to any real world situation depends upon how precisely the parameters and values of our theoretical reconstruction of a real world approximate reality. At this stage, however, I find it difficult to see how the heavily feared ‘subjectivity’ can be avoided. Simply put, plug in different values into the theorem and you’ll get a different answer. How does one decide which value to plug in?
You don’t have to do very much research to discover that Bayes’ Theorem does not fear subjectivity; it welcomes it. Subjective probability is built into the process. And you say you’re not sure about what value to plug in for prior probability? Then guess! No, really, it’s OK. What’s that? You don’t even have a good guess? Then plug in 50% and proceed.
It’s Bayes’ casual embrace of uncertainty and subjectivity — its treatment of subjective prior probability (degree of belief) — that drives the frequentists crazy. However, the results speak for themselves.
And as far as getting different answers when you plug in different numbers, that’s a common feature in equations. Stick in a different mass value in F = ma, and — boom! — you get a different value for force. It’s like magic! Good grief. What do they teach at Cambridge these days?
The proper application of BT forces us to estimate the prior probabilities. It encourages us to quantify elements that we might not have even considered in the past. It takes into account our degree of belief about a subject. And it makes us apply mathematical rigor to topics we used to think could be understood only through intuition. Hence BT’s imposed discipline is extraordinarily useful, since we can now haggle over the inputs (that’s why they’re called variables) rather than argue over intuitive conclusions about plausibility — because truthfully, when a scholar writes something like “Nobody would ever make that up,” it’s nothing but an untested assertion.
Bayes’ Theorem ascendant
If you can possibly spare the time, please watch the video after the page break. In it, Sharon Bertsch McGrayne, author of The Theory That Would Not Die: How Bayes’ Rule Cracked the Enigma Code, Hunted Down Russian Submarines, and Emerged Triumphant from Two Centuries of Controversy recounts the story of how Bayes’ Theorem won the day. She tells us how BT is well suited for situations with extremely limited historical data or even no historical data — e.g., predicting the probability of the occurrence of an event that has never happened before.
[youtube=http://www.youtube.com/watch?v=oTltncUkckQ]
It’s frustrating that the video cuts off her answer to the last question. However, I hope this short introduction helps anyone who was misled by Hoffmann’s comments. BT can be used in many situations, even those in which there is a limited amount of data, prone to subjectivity and uncertainty, to arrive at statistically meaningful results.
Currently, BT is making headway in forensics and in the courtroom, problem domains not too terribly different from historical research. Is it controversial? Yes, of course. Bayes’ Theorem has a long history of early rejection each time it’s introduced to a new realm. But eventually, it proves itself undeniably useful, and finally irresistible. Can it “prove history”? Time will tell, but I wouldn’t bet against Bayes.
Tim Widowfield
Latest posts by Tim Widowfield (see all)
- How Did We Get Here? Part 2: Belief - 2024-12-30 21:31:05 GMT+0000
- How Did We Get Here? Part 1 - 2024-11-21 01:22:29 GMT+0000
- What Did Marx Say Was the Cause of the American Civil War? (Part 1) - 2024-05-12 19:09:26 GMT+0000
If you enjoyed this post, please consider donating to Vridar. Thanks!
“I’ll see your Jesus denial and raise you Bayes’ theorem denial!” Such a stupid conversation.
I am currently about ¾ of the way through The Theory That Would Not Die right now. It is a very informative and well-written survey of the history of Bayesian theory and methodology.
Curiously, Hoffman, by implying that BT’s usefulness is limited to instances where there are previous verifiable occurrences, actually betrays his ignorance of the difference between a “frequentist” approach to probability and a Bayesian one. He misrepresents BT as though these two methods are equivalent when they are actually diametrically opposed.
It would be kinda cute if he wasn’t so smug in his certitude about it.
People who criticize Bayes’ formula are not actually objecting to the theorem, but rather the interpretation of probability. Essentially, Hoffman is objecting to the notion that we can talk about the probability of something having occurred in the past. In other words, he thinks that statements such as “Julius Caesar probably crossed the Rubicon,” “the resurrection probably occurred,” or “it probably didn’t rain in Phoenix yesterday” are meaningless. That’s the only way to make sense of his quotes.
Malcolm: “Julius Caesar probably crossed the Rubicon,” “the resurrection probably occurred,” or “it probably didn’t rain in Phoenix yesterday”
In fact, one can apply BT to all three of the examples you cite above.
Try it. It works.
Merely asserting that assessing their respective probabilities methodologically is “meaningless” would just be a form of arrogant evasiveness.
Of course I know that. My point is that to apply BT to those situations, one first has to agree that they concern the concept of probability at all. There are those (strict frequentists) who will actually insist that they don’t. I don’t think that it is necessarily evasiveness on their part but rather philosophical differences. In Hoffmann’s case, however, it just seems that he’s looking for any excuse to criticize Carrier, whether he really believes it or not, as my quote of Hoffmann below demonstrates.
“How can it be useful in determining whether events ”actually” transpired in the past, that is, when the sample field itself consists of what has already occurred (or not occurred) and when B is the probability of it having happened?”
Statements like this (and its ilk; there are at least 3 of them in Hoffman’s quotes) demonstrate a complete lack of understanding of both probability and Bayes’ theorem. Here’s a real-world, routine application of Bayes’ theorem in medicine (it was in my probability textbook in college, although the disease wasn’t specified): Let’s say 1% of the population is HIV+. Furthermore, HIV antibody tests have a 1% false positive rate (which used to be true, but now it’s much lower) and a 0.1% false negative rate (this number is not so important). If you take an HIV test and the result is positive, what is the probability that you actually have the disease? Using Bayes’ theorem, one gets around 50%. Note that we’re not talking about future possibilities here – you either have been infected already or you haven’t. This application is quite common and standard.
Now, one can argue that one should be looking at the entire population but rather a subset that matches some other characteristic of you (whether you use IV drugs, have unprotected sex, etc.), but that is a separate issue – the reference-class problem – that plagues all meaningful interpretations of probability, especially the frequentist one. It has nothing to do with Bayes’ theorem per se. BT is just a straightforward formula derived from the axioms and definitions of mathematical probability theory – it can used in any situation where one has probabilities .
Another example of a typical and simple application of Bayes’ theorem to a situation where the event occurred in the past is the Monty Hall problem. A the time where you have to make your choice, what is behind each door is already determined.
The Mony Hall Problem: http://en.wikipedia.org/wiki/Monty_Hall_problem
Upon reading Hoffmann’s entire essay, I see that he is confusing the applicability of BT with its usefulness in practice. He even says this at one point: ‘Historical argumentation is both non-intuitive and probabilistic (in the sense of following the “law of likelihood”); but tends to favor the view that Bayes’s excessive use of “prior possibilities” are subjective and lack probative force.’ Leaving aside the ambiguity of this sentence (what tends to favor?), he admits that historical argumentation is probabilistic. Since BT is a theorem in probability, it can therefore be applied to historical argumentation.
Apparently, his only real objection is that prior probabilities are subjective. But BT forces one to spell them out, and then one can argue about the values for them. In fact, since prior probabilities are themselves conditional on background information, one can derive them from a series of other applications of BT from more “fundamental” priors. Eventually you hopefully reach prior probabilities that almost everyone can agree on, for example, those that come from games of chance or repeatable experiments. Even if one doesn’t, at the very least your assumptions are made more clear.
Maybe it’s just me, but reading this I immediately thought of Hoffman’s own writing style…
It isn’t just you. Some parts of “Controversy, Mythicism, and the Historical Jesus” left me “cowed.”
Here’s a Hoffmannogram that left me breathless (and nearly comatose):
I haven’t had to process a sentence like that since I read Absalom, Absalom!.
It’s a John Norman Run-On Sentence! Why waste a period? They cost money. Use one period per paragraph and think of the savings!
You couldn’t have picked a better example. There are many others, but this one is good enough.
From the very start you’re left wondering what Hoffmann’s really talking about.
First “the purposes of the gospels” and then “the worldview of the gospels” are brought into opposition, with a declaration of “tension”. But is that truly clear? How come the “worldview” is not included in “the purposes of the gospels”?
He wants to refer to distinct aspects of the gospel propaganda, but without being explicit and clarifying them, preferring to keep them mingled on purpose.
Then he continues with a kind of incantation that tends to leave your brain numb and tired from the effort of following the thread, if there is one.
Then you have all the undermining or discounting words such as “perhaps”, “cannot have been”, “not necessarily,”seems to have”, creating a confused image where everything becomes possible.
If you stop to take a breath, you start wondering about “friendly to the more mundane aspects of tradition”. Why can’t he speak like a straightforward Protestant scholar who says black is black and white is white? Because he can’t. He sees everything in an ambiguous shade of gray, and that is the impression he wants to create, blurring the sharp outlines of concepts and distinctions.
What do we learn from reading his stuff? Is it a waste of time?
One of the most fundamental aspects of science, falsifiability, follows directly from Bayes Theorem. If BT doesn’t apply to history, then it has to follow that any historical hypothesis is unfalsifiable.
I posted this following comment on Hoffmann’s blog, but it got disappeared by the Internet and my crappy browser. So I’ll post it here:
If probability theory only applied “to future events” then there wouldn’t be a name for a misunderstanding of probability theory in court trials, which necessarily deal with past events. I’m not aware of any definition of probability theory that says it only applies to future events. It applies to incomplete information (I suggest everyone read that link. In normal language if we use terms like “x hypothesis is more likely than y hypothesis” this is necessarily mathematical language and can only make sense if expressed numerically).
But Hoffmann’s post seems to be arguing that Bayesianism is only about ontological or objective probability and not epistemic or subjective probability. This is part of the ongoing debate between Frequentism and Bayesianism, which for now is unresolved. Frequentists generally think that probabilities are inherent properties of objects or experiments (ontological). So if we have 95% confidence in some experimental outcome, and if you run that experiment 100 times, 95 of the experiemnts run should give the same result. 95% is an inherent property of the experiment. Or, a fair coin inherently has a 50% chance of landing heads because that is the definition of a fair coin. You can continue to flip a coin in a succession of experiments and it will regress towards the mean of 50%. This might explain the accusation of attempting mathematical precision; mathematical precision only applies to ontological probability.
But we can also talk about epistemic probability, or how much confidence an individual has in some hypothesis or idea. This is one reason why Frequentists accuse Bayesians of being too subjective. So for example, in the study I posted we had this scenario:
If we were only talking about ontological probability, then telling students to rank these options by how likely they are would make no sense. In reality, Linda ontologically is either a bank teller or she isn’t (this is how Swinburne failed in the example). She isn’t 80% bank teller. But we can still have some sort of epistemic warrant for believing that she is/isn’t; we can give a number for how likely it is — based on our own personal experiences — that she is a bank teller. We should be able to translate “I think it is highly probable that Linda is a bank teller” to “I have 80% confidence that Linda is a bank teller” (based on the first link I posted).
Of course, this experiment is an example of why Occam’s Razor makes sense. OR follows from probability theory; Linda being a bank teller and a feminist is less likely than her just being a feminist. Even though that doesn’t make intuitive sense, it is the “simpler” hypothesis.
Overall, if you take only a Frequentist view of probability, then attempting to use probability theory in historical analysis might not make sense. Yet there seem to be some Frequentist applications to historical questions. But if, for example, one of Hoffmann’s students missed class he would probably conclude that it was more likely that the student was sick or goofing off instead of having been kidnapped by aliens. If he agrees with that reasoning, he has just used Bayes Theorem!
J. Quinton:
Your post got through or was reposted, on Hoffmann’s blog. CHeck it out: Hoffmann is interested and commenting; and asking you to follow up with clarifications.
I posted this following comment on Hoffmann’s blog, but it got disappeared by the Internet and my crappy browser. So I’ll post it here:
If probability theory only applied “to future events” then there wouldn’t be a name for a misunderstanding of probability theory in court trials, which necessarily deal with past events. I’m not aware of any definition of probability theory that says it only applies to future events. It applies to incomplete information (I suggest everyone read that link. In normal language if we use terms like “x hypothesis is more likely than y hypothesis” this is necessarily mathematical language and can only make sense if expressed numerically).
But Hoffmann’s post seems to be arguing that Bayesianism is only about ontological or objective probability and not epistemic or subjective probability. This is part of the ongoing debate between Frequentism and Bayesianism, which for now is unresolved. Frequentists generally think that probabilities are inherent properties of objects or experiments (ontological). So if we have 95% confidence in some experimental outcome, and if you run that experiment 100 times, 95 of the experiemnts run should give the same result. 95% is an inherent property of the experiment. Or, a fair coin inherently has a 50% chance of landing heads because that is the definition of a fair coin. You can continue to flip a coin in a succession of experiments and it will regress towards the mean of 50%. This might explain the accusation of attempting mathematical precision; mathematical precision only applies to ontological probability.
But we can also talk about epistemic probability, or how much confidence an individual has in some hypothesis or idea. This is one reason why Frequentists accuse Bayesians of being too subjective. So for example, in the study I posted we had this scenario:
If we were only talking about ontological probability, then telling students to rank these options by how likely they are would make no sense. In reality, Linda ontologically is either a bank teller or she isn’t (this is how Swinburne failed in the example). She isn’t 80% bank teller. But we can still have some sort of epistemic warrant for believing that she is/isn’t; we can give a number for how likely it is — based on our own personal experiences — that she is a bank teller. We should be able to translate “I think it is highly probable that Linda is a bank teller” to “I have 80% confidence that Linda is a bank teller”.
Of course, this experiment is an example of why Occam’s Razor makes sense. OR follows from probability theory; Linda being a bank teller and a feminist is less likely than her just being a feminist… even though that doesn’t make intuitive sense.
Overall, if you take only a Frequentist view of probability, then attempting to use probability theory in historical analysis might not make sense. Yet there seem to be some Frequentist applications to historical questions. But if, for example, one of Hoffmann’s students missed class he would probably conclude that it was more probable that the student was sick or goofing off instead of having been kidnapped by aliens. If he agrees with that reasoning, he has just used Bayes Theorem!